This is the multi-page printable view of this section. Click here to print.
Blog
- Blogs
- The OpenFunction TOC Officially Established!
- Elastic Kubernetes Log Alerts with OpenFunction and Kafka
- New Releases
- OpenFunction 1.2.0: integrating KEDA http-addon as a synchronous function runtime
- Announcing OpenFunction 1.1.0: Support Dapr State Management and Refactor Function Triggers
- Announcing OpenFunction 1.0.0: Integrate WasmEdge to support Wasm Functions and Enhanced CI/CD
- Announcing OpenFunction 0.8.0: Speed up function launching with Dapr Proxy
- Announcing General Availability of OpenFunction 0.7.0
- Announcing OpenFunction 0.6.0: FaaS observability, HTTP trigger, and more
- Release v0.4.0
- Release v0.3.1
- Release v0.3.0
- Release v0.2.0
- Release v0.1.0
Blogs
The OpenFunction TOC Officially Established!
In recent days, OpenFunction has officially become a sandbox project of Cloud Native Computing Foundation (CNCF), opening a new chapter of the open-source project that is 100% powered by the community. To better support project development and maintain project neutrality, we have established the OpenFunction Technical Oversight Committee (TOC). With the primary objective of overseeing the technical vision of OpenFunction, the OpenFunction Steering Committee established the TOC. The technical vision of the project identifies technical orientation, technology roadmapping, architecture design, management, and promotion.
The TOC expects to hold biweekly meetings on Tuesday afternoon after the community meetings of OpenFunction. The meetings notes are accessible to all. We appreciate the open conversation and proposals all around.
Roles
The TOC works as a technical gatekeeper for the project and provides overall technical guidelines for special interest groups (SIGs) and working groups. Specifically, the TOC assumes the following roles:
- Determine the overall technical orientation and design of the project.
- Create a roadmap for the project.
- Approve the creation and dissolution of SIGs and elect SIG leaders.
- Approve the management operations on GitHub repositories related to the project, for example, the operations to create or delete repositories.
- Resolve issues raised by SIGs and working groups.
Members
The following table lists the members of the TOC in alphabetical order.
Member | Company | GitHub ID | Join Date | |
---|---|---|---|---|
![]() | Benjamin Huo | QingCloud | @benjaminhuo | Since the project was initiated |
![]() | Geff Zhang | Weyhd | @geffzhang | 2022-06-06 |
![]() | Haili Zhang | UISEE | @webup | 2022-06-06 |
![]() | Lize Cai | SAP | @lizzcai | 2022-06-06 |
![]() | Tian Fang | StreamNative | @tpiperatgod | 2022-06-06 |
![]() | Wanjun Lei | QingCloud | @wanjunlei | 2022-06-06 |
![]() | Wei Zhang | Boban Data | @arugal | 2022-06-06 |
Elastic Kubernetes Log Alerts with OpenFunction and Kafka
Overview
How do you handle container logs collected by the message server? You may face a dilemma: Deploying a dedicated log processing workload can be costly, and it is difficult to assess the number of standby log processing workloads required when the quantity of logs fluctuates sharply. This blog post offers ideas for serverless log processing, which reduces the link cost while improving flexibility.
Our general design idea is to add a Kafka server as a log receiver, and then use the log input to the Kafka server as an event to drive the serverless workloads to handle logs. Roughly, the following steps are involved:
- Set up a Kafka server as the log receiver for Kubernetes clusters.
- Deploy OpenFunction to provide serverless capabilities for log processing workloads.
- Write log processing functions to grab specific logs to generate alerting messages.
- Configure Notification Manager to send alerts to Slack.
In this scenario, we will make use of the serverless capabilities ofOpenFunction.
OpenFunction is an open-source FaaS (serverless) project initiated by the KubeSphere community. It is designed to allow users to focus on their business logic without the hassle of caring about the underlying operating environment and infrastructure. Currently, the project provides the following key capabilities:
- Builds OCI images from Dockerfile or Buildpacks.
- Runs serverless workloads using Knative Serving or Async (backed by KEDA + Dapr) as a runtime.
- Equipped with a built-in event-driven framework.
Use Kafka as a Log Receiver
First, enable the logging component for the KubeSphere platform (For more information, please refer toEnable Pluggable Components. Next, we can use strimzi-kafka-operator to build a minimal Kafka server.
In the
default
namespace, install strimzi-kafka-operator.helm repo add strimzi https://strimzi.io/charts/ helm install kafka-operator -n default strimzi/strimzi-kafka-operator
Run the following commands to create a Kafka cluster and a Kafka topic in the
default
namespace. The storage type of the created Kafka and ZooKeeper clusters is ephemeral. Here, we useemptyDir
for demonstration.Note that we have created a topic named
logs
for follow-up use.cat <<EOF | kubectl apply -f - apiVersion: kafka.strimzi.io/v1beta2 kind: Kafka metadata: name: kafka-logs-receiver namespace: default spec: kafka: version: 2.8.0 replicas: 1 listeners: - name: plain port: 9092 type: internal tls: false - name: tls port: 9093 type: internal tls: true config: offsets.topic.replication.factor: 1 transaction.state.log.replication.factor: 1 transaction.state.log.min.isr: 1 log.message.format.version: '2.8' inter.broker.protocol.version: "2.8" storage: type: ephemeral zookeeper: replicas: 1 storage: type: ephemeral entityOperator: topicOperator: {} userOperator: {} --- apiVersion: kafka.strimzi.io/v1beta1 kind: KafkaTopic metadata: name: logs namespace: default labels: strimzi.io/cluster: kafka-logs-receiver spec: partitions: 10 replicas: 3 config: retention.ms: 7200000 segment.bytes: 1073741824 EOF
Run the following command to check the Pod’s status and wait until Kafka and ZooKeeper runs and starts.
$ kubectl get po NAME READY STATUS RESTARTS AGE kafka-logs-receiver-entity-operator-568957ff84-nmtlw 3/3 Running 0 8m42s kafka-logs-receiver-kafka-0 1/1 Running 0 9m13s kafka-logs-receiver-zookeeper-0 1/1 Running 0 9m46s strimzi-cluster-operator-687fdd6f77-cwmgm 1/1 Running 0 11m
Run the following command to view metadata of the Kafka cluster:
# Starts a utility pod. $ kubectl run utils --image=arunvelsriram/utils -i --tty --rm # Checks metadata of the Kafka cluster. $ kafkacat -L -b kafka-logs-receiver-kafka-brokers:9092
Add this Kafka server as a log receiver.
Log in to the web console of KubeSphere as admin. In the upper-left corner, choose Platform > Cluster Management.
If you have enabled the multi-cluster feature, you need to select a cluster.
On the Cluster Management page, click Log Collections under Cluster Settings.
Click Add Log Receiver, and then click Kafka. Enter the service address and port number of Kafka, and then click OK.
Run the following commands to verify that Kafka clusters can collect logs from Fluent Bit.
# Starts a utility pod. $ kubectl run utils --image=arunvelsriram/utils -i --tty --rm # Checks logs in the `logs` topic $ kafkacat -C -b kafka-logs-receiver-kafka-0.kafka-logs-receiver-kafka-brokers.default.svc:9092 -t logs
Deploy OpenFunction
According to the design in Overview, we need to deploy OpenFunction first. As OpenFunction has referenced multiple third-party projects, such as Knative, Tekton, ShipWright, Dapr, and KEDA, it is cumbersome if you manually deploy it. It is recommended that you refer to Prerequisites to quickly deploy dependencies of OpenFunction.
In the command,
--with-shipwright
means that Shipwright is deployed as the build driver for the function;--with-openFuncAsync
means that OpenFuncAsync Runtime is deployed as the load driver for the function. When you have limited access to GitHub and Google, you can add the--poor-network
parameter to download related components.
sh hack/deploy.sh --with-shipwright --with-openFuncAsync --poor-network
Deploy OpenFunction.
We install the latest stable version here. Alternatively, you can use the development version. For more information, please refer to theInstall OpenFunction section.
To make sure that Shipwright works properly, we provide a default build policy, and you can run the following commands to set the policy.
kubectl apply -f https://raw.githubusercontent.com/OpenFunction/OpenFunction/main/config/strategy/openfunction.yaml
kubectl apply -f https://github.com/OpenFunction/OpenFunction/releases/download/v0.3.0/bundle.yaml
Write a Log Processing Function
In this example, we install WordPress as the log producer. The application’s workload resides in the demo-project
namespace and the Pod’s name is wordpress-v1-f54f697c5-hdn2z
.
When a request returns 404, the log content is as follows:
{"@timestamp":1629856477.226758,"log":"*.*.*.* - - [25/Aug/2021:01:54:36 +0000] \"GET /notfound HTTP/1.1\" 404 49923 \"-\" \"curl/7.58.0\"\n","time":"2021-08-25T01:54:37.226757612Z","kubernetes":{"pod_name":"wordpress-v1-f54f697c5-hdn2z","namespace_name":"demo-project","container_name":"container-nrdsp1","docker_id":"bb7b48e2883be0c05b22c04b1d1573729dd06223ae0b1676e33a4fac655958a5","container_image":"wordpress:4.8-apache"}}
Here are our needs: When a request returns 404, the Notification Manager sends a notification to the receiver (Configure a Slack alert receiver according to Configure Slack Notifications, and records the namespace, Pod name, request path, request method, and other information. Therefore, we write a simple function:
You can learn how to use
openfunction-context
from OpenFunction Context Spec, which is a tool library provided by OpenFunction for writing functions. You can learn more about OpenFunction functions from OpenFunction Samples.
package logshandler
import (
"encoding/json"
"fmt"
"log"
"regexp"
"time"
ofctx "github.com/OpenFunction/functions-framework-go/openfunction-context"
alert "github.com/prometheus/alertmanager/template"
)
const (
HTTPCodeNotFound = "404"
Namespace = "demo-project"
PodName = "wordpress-v1-[A-Za-z0-9]{9}-[A-Za-z0-9]{5}"
AlertName = "404 Request"
Severity = "warning"
)
// The ctx parameter of the LogHandler function provides a context handle for user functions in the cluster. For example, ctx.SendTo is used to send data to a specified destination.
// The in parameter in the LogsHandle function is used to pass byte data (if any) from the input to the function.
func LogsHandler(ctx *ofctx.OpenFunctionContext, in []byte) int {
content := string(in)
// We set three regular expressions here for matching the HTTP status code, resource namespace, and Pod name of resources, respectively.
matchHTTPCode, _ := regexp.MatchString(fmt.Sprintf(" %s ", HTTPCodeNotFound), content)
matchNamespace, _ := regexp.MatchString(fmt.Sprintf("namespace_name\":\"%s", Namespace), content)
matchPodName := regexp.MustCompile(fmt.Sprintf(`(%s)`, PodName)).FindStringSubmatch(content)
if matchHTTPCode && matchNamespace && matchPodName != nil {
log.Printf("Match log - Content: %s", content)
// If the input data matches all three regular expressions above, we need to extract some log information to be used in the alert.
// The alert contains the following information: HTTP method of the 404 request, HTTP path, and Pod name.
match := regexp.MustCompile(`([A-Z]+) (/\S*) HTTP`).FindStringSubmatch(content)
if match == nil {
return 500
}
path := match[len(match)-1]
method := match[len(match)-2]
podName := matchPodName[len(matchPodName)-1]
// After we collect major information, we can use the data struct of altermanager to compose an alert.
notify := &alert.Data{
Receiver: "notification_manager",
Status: "firing",
Alerts: alert.Alerts{},
GroupLabels: alert.KV{"alertname": AlertName, "namespace": Namespace},
CommonLabels: alert.KV{"alertname": AlertName, "namespace": Namespace, "severity": Severity},
CommonAnnotations: alert.KV{},
ExternalURL: "",
}
alt := alert.Alert{
Status: "firing",
Labels: alert.KV{
"alertname": AlertName,
"namespace": Namespace,
"severity": Severity,
"pod": podName,
"path": path,
"method": method,
},
Annotations: alert.KV{},
StartsAt: time.Now(),
EndsAt: time.Time{},
GeneratorURL: "",
Fingerprint: "",
}
notify.Alerts = append(notify.Alerts, alt)
notifyBytes, _ := json.Marshal(notify)
// Use ctx.SendTo to send the content to the "notification-manager" output (you can find its definition in the following logs-handler-function.yaml function configuration file.
if err := ctx.SendTo(notifyBytes, "notification-manager"); err != nil {
panic(err)
}
log.Printf("Send log to notification manager.")
}
return 200
}
Upload this function to the code repository and record the URL of the code repository and the path of the code in the repository, which will be used in the Create a function step.
You can find this case in OpenFunction Samples.
Create a Function
Use OpenFunction to build the above function. First, set up a key file push-secret
to access the image repository (After the OCI image is constructed using the code, OpenFunction will upload the image to the image repository for subsequent load startup.):
REGISTRY_SERVER=https://index.docker.io/v1/ REGISTRY_USER=<your username> REGISTRY_PASSWORD=<your password>
kubectl create secret docker-registry push-secret \
--docker-server=$REGISTRY_SERVER \
--docker-username=$REGISTRY_USER \
--docker-password=$REGISTRY_PASSWORD
Apply the function configuration file logs-handler-function.yaml
.
The function definition explains the use of two key components:
Dapr shields complex middleware from applications, making it easy for the
logs-handler
function to handle Kafka events.KEDA drives the startup of the
logs-handler
function by monitoring event traffic in the message server, and dynamically extends thelogs-handler
instance based on the consumption delay of Kafka messages.
apiVersion: core.openfunction.io/v1alpha1
kind: Function
metadata:
name: logs-handler
spec:
version: "v1.0.0"
# Defines the upload path for the built image.
image: openfunctiondev/logs-async-handler:v1
imageCredentials:
name: push-secret
build:
builder: openfunctiondev/go115-builder:v0.2.0
env:
FUNC_NAME: "LogsHandler"
# Defines the path of the source code.
# url specifies the URL of the above-mentioned code repository.
# sourceSubPath specifies the path of the code in the repository.
srcRepo:
url: "https://github.com/OpenFunction/samples.git"
sourceSubPath: "functions/OpenFuncAsync/logs-handler-function/"
serving:
# OpenFuncAsync is an event-driven, asynchronous runtime implemented in OpenFunction by using KEDA_Dapr.
runtime: "OpenFuncAsync"
openFuncAsync:
# This section defines the function input (kafka-receiver) and the output (notification-manager), which correspond to definitions in the components section.
dapr:
inputs:
- name: kafka-receiver
type: bindings
outputs:
- name: notification-manager
type: bindings
params:
operation: "post"
type: "bindings"
annotations:
dapr.io/log-level: "debug"
# This section defines the above-mentioned input and output (that is, Dapr Components).
components:
- name: kafka-receiver
type: bindings.kafka
version: v1
metadata:
- name: brokers
value: "kafka-logs-receiver-kafka-brokers:9092"
- name: authRequired
value: "false"
- name: publishTopic
value: "logs"
- name: topics
value: "logs"
- name: consumerGroup
value: "logs-handler"
# This is the URL of KubeSphere notification-manager.
- name: notification-manager
type: bindings.http
version: v1
metadata:
- name: url
value: http://notification-manager-svc.kubesphere-monitoring-system.svc.cluster.local:19093/api/v2/alerts
keda:
scaledObject:
pollingInterval: 15
minReplicaCount: 0
maxReplicaCount: 10
cooldownPeriod: 30
# This section defines the trigger of the function, that is, the log topic of the Kafka server.
# This section also defines the message lag threshold (the value is 10), which means that when the number of lagged messages exceeds 10, the number of logs-handler instances will automatically scale out.
triggers:
- type: kafka
metadata:
topic: logs
bootstrapServers: kafka-logs-receiver-kafka-brokers.default.svc.cluster.local:9092
consumerGroup: logs-handler
lagThreshold: "10"
Demonstrate the Result
Disable the Kafka log receiver first: On the Log Collections page, click Kafka to go to the details page, and choose More > Change Status > Close.
Wait for a while, and then it can be observed that number of instances of the logs-handler
function has reduced to 0.
Then set the status of the Kafka log receiver to Collecting, and logs-handler
also starts.
~# kubectl get po --watch
NAME READY STATUS RESTARTS AGE
kafka-logs-receiver-entity-operator-568957ff84-tdrrx 3/3 Running 0 7m27s
kafka-logs-receiver-kafka-0 1/1 Running 0 7m48s
kafka-logs-receiver-zookeeper-0 1/1 Running 0 8m12s
logs-handler-serving-kpngc-v100-zcj4q-5f46996f8c-b9d6f 2/2 Terminating 0 34s
strimzi-cluster-operator-687fdd6f77-kc8cv 1/1 Running 0 10m
logs-handler-serving-kpngc-v100-zcj4q-5f46996f8c-b9d6f 2/2 Terminating 0 36s
logs-handler-serving-kpngc-v100-zcj4q-5f46996f8c-b9d6f 0/2 Terminating 0 37s
logs-handler-serving-kpngc-v100-zcj4q-5f46996f8c-b9d6f 0/2 Terminating 0 38s
logs-handler-serving-kpngc-v100-zcj4q-5f46996f8c-b9d6f 0/2 Terminating 0 38s
logs-handler-serving-kpngc-v100-zcj4q-5f46996f8c-9kj2c 0/2 Pending 0 0s
logs-handler-serving-kpngc-v100-zcj4q-5f46996f8c-9kj2c 0/2 Pending 0 0s
logs-handler-serving-kpngc-v100-zcj4q-5f46996f8c-9kj2c 0/2 ContainerCreating 0 0s
logs-handler-serving-kpngc-v100-zcj4q-5f46996f8c-9kj2c 0/2 ContainerCreating 0 2s
logs-handler-serving-kpngc-v100-zcj4q-5f46996f8c-9kj2c 1/2 Running 0 4s
logs-handler-serving-kpngc-v100-zcj4q-5f46996f8c-9kj2c 2/2 Running 0 11s
Next, initialize a request for a non-existent path of the WordPress application:
curl http://<wp-svc-address>/notfound
You can see that Slack has received this message (Slack will not receive an alert message when we visit the WordPress site properly).
Explore More Possibilities
We can further discuss a solution using synchronous functions:
To use Knative Serving properly, we need to set the load balancer address of its gateway. (You can use the local address as a workaround.)
# Repalce the following "1.2.3.4" with the actual values.
$ kubectl patch svc -n kourier-system kourier \
-p '{"spec": {"type": "LoadBalancer", "externalIPs": ["1.2.3.4"]}}'
$ kubectl patch configmap/config-domain -n knative-serving \
-type merge --patch '{"data":{"1.2.3.4.sslip.io":""}}'
OpenFunction drives the running of the Knative
function in two ways: (1) Use the Kafka server in asynchronous mode; (2) Use its own event framework to connect to the Kafka server, and then operate in Sink mode. You can refer to the case in OpenFunction Samples.
In this solution, the processing speed of synchronous functions is lower than that of asynchronous functions. We can also use KEDA to trigger the concurrency mechanism of Knative Serving, but it is not as convenient as asynchronous functions. (In the future, we will optimize the OpenFunction event framework to make up for the shortcomings of synchronous functions.)
It can be seen that different types of serverless functions have their unique advantages depending on task scenarios. For example, when it comes to handling an orderly control flow function, a synchronous function outperforms an asynchronous function.
Summary
Serverless matches our expectations for rapid disassembly and reconstruction of business scenarios.
As you can see in this case, OpenFunction not only increases flexibility of log processing and alert notification links by using the serverless technology, but also uses a function framework to simplify complex setups typically required to connect to Kafka into semantically clear code. Moreover, we are also continuously developing OpenFunction so that components can be powered by our own serverless capabilities in follow-up releases.
New Releases
OpenFunction 1.2.0: integrating KEDA http-addon as a synchronous function runtime
OpenFunction is an open-source cloud-native FaaS (Function as a Service) platform designed to help developers focus on their business logic. We are thrilled to announce another important update for OpenFunction, the release of version v1.2.0!
In this update, we continue to strive to provide developers with more flexible and powerful tools, and have added some new features. This version integrates KEDA http-addon as a synchronous function runtime, supports adding environment variables when enabling SkyWalking tracing, and supports recording build time. Additionally, several components have been upgraded and multiple bugs have been fixed.
Here are the main highlights of this version update:
integrating KEDA http-addon as a synchronous function runtime
KEDA http-addon is an additional component of KEDA that automatically scales HTTP servers based on changes in HTTP traffic, including scaling from zero to handle traffic and scaling down to zero when there is no traffic.
The working principle of KEDA http-addon is that it creates a component called Interceptor in the Kubernetes cluster to receive all HTTP requests and forward them to the target application. At the same time, it reports the length of the request queue to a component called External Scaler, which triggers KEDA’s automatic scaling mechanism. This allows your HTTP application to dynamically adjust the number of replicas based on the actual traffic demand.
In OpenFunction version v1.2.0, we have integrated KEDA http-addon as an option for synchronous function runtime. This means that you can use OpenFunction to create and manage HTTP-based functions and leverage the capabilities of KEDA http-addon for efficient and flexible elastic scaling. To deploy and run your HTTP functions, you simply need to specify the value of serving.triggers[*].http.engine
as “keda” when creating the Function resource and configure the relevant parameters of keda.httpScaledObject
in serving.scaleOptions
.
Support for recording events when the states of Function, Builder, and Serving change
Events are an important resource type in Kubernetes that can record important or interesting occurrences within a cluster. Events can help users and developers understand changes in the state of resources within the cluster and handle any abnormalities accordingly.
In OpenFunction version v1.2.0, we support recording events when the states of Function, Builder, and Serving change. This allows you to gain more information about what happens during the function building and running processes by reviewing the events. For example, you can see events such as the start, end, or failure of function building, as well as events related to the creation, update, or deletion of function runtimes.
Other improvements and optimizations
In addition to the major changes mentioned above, this version also includes the following modifications and enhancements:
- Upgraded KEDA to v2.10.1 and HPA (Horizontal Pod Autoscaler) API version to v2, improving stability and compatibility.
- Added support for recording build time, allowing you to track the duration of function builds.
- Adjusted the CI (Continuous Integration) process and fixed some minor issues.
- Fixed a bug in the keda http-addon runtime that caused functions to not run properly.
- Upgraded several components in the charts, including keda, dapr, and contour, to ensure the use of the latest versions and features.
- These are the main functional changes in OpenFunction v1.2.0. We would like to express our sincere gratitude to all contributors for their participation and contributions.
To learn more about OpenFunction and this version update, please visit our official website and GitHub page.
- Official Website:https://openfunction.dev/
- Github:https://github.com/OpenFunction/OpenFunction/releases/tag/v1.2.0
Announcing OpenFunction 1.1.0: Support Dapr State Management and Refactor Function Triggers
OpenFunction is a cloud-native open-source FaaS (Function as a Service) platform aiming to let you focus on your business logic only. Today, we are thrilled to announce the general availability of OpenFunction 1.1.0.
In this release, we have added the v1beta2 API and support Dapr State management. In addition, we enhanced some features and fixed bugs, making OpenFunction more stable and easy to use.
The following introduces the major updates.
Add the v1beta2 API
In this release, we have added the v1beta2 API. The v1beta1 API has been deprecated and will be removed. You can learn more about the v1beta2 API from this proposal.
Support Dapr state management
Previously, OpenFunction supports the pub/sub and bindings building blocks, and state management is a building block that is useful for stateful functions. With the use of state store components, you can build functions with persistent state, allowing them to save and restore their own states.
You can define state stores in Function CR, and OpenFunction will manage the corresponding Dapr components.
The functions can use the encapsulated state management API of Dapr to save, read, and query key/value pairs in the defined state storage.
Refactor function triggers
Previously, we use runtime: knative
and runtime: async
to distinguish sync and async functions, which is sort of difficult. Actually the difference between sync and async functions lies in the trigger type:
Sync functions are triggered by
HTTP
events, which are defined by specifyingruntime: knative
.Async functions are triggered by events from components of
Dapr bindings
orDapr pubsub
.runtime: async
andinputs
have to be used together to specify triggers for async functions.
Now we use triggers
to replace runtime
and inputs
.
HTTP Trigger
triggers a function with an HTTP request. You can define an HTTP Trigger
for a function like this:
apiVersion: core.openfunction.io/v1beta2
kind: Function
metadata:
name: function-sample
spec:
serving:
triggers:
http:
port: 8080
route:
rules:
- matches:
- path:
type: PathPrefix
value: /echo
Dapr Trigger
triggers a function with events from Dapr bindings
or Dapr pubsub
. You can define a function with Dapr Trigger
like this:
apiVersion: core.openfunction.io/v1beta2
kind: Function
metadata:
name: logs-async-handler
namespace: default
spec:
serving:
bindings:
kafka-receiver:
metadata:
- name: brokers
value: kafka-server-kafka-brokers:9092
- name: authRequired
value: "false"
- name: publishTopic
value: logs
- name: topics
value: logs
- name: consumerGroup
value: logs-handler
type: bindings.kafka
version: v1
triggers:
dapr:
- name: kafka-receiver
type: bindings.kafka
Other enhancements
- Delete the
lastTransitionTime
field from the gateway status to prevent frequent triggering of reconcile. - Allow to set scopes when creating Dapr components.
- Support the ability to set cache images to improve build performance when using OpenFunction strategies.
- Support the ability to set bash images of OpenFunction strategies.
These are the main feature changes in OpenFunction v1.1.0 and we would like to thank all contributors for your contributions. If you are looking for an efficient and flexible cloud-native function development platform, OpenFunction v1.1.0 is the perfect choice for you.
For more details and documentation, please visit our website and GitHub repo.
- Official Website: https://openfunction.dev/
- Github:https://github.com/OpenFunction/OpenFunction/releases/tag/v1.1.0
Announcing OpenFunction 1.0.0: Integrate WasmEdge to support Wasm Functions and Enhanced CI/CD
OpenFunction is a cloud-native open-source FaaS (Function as a Service) platform aiming to let you focus on your business logic only. Today, we are thrilled to announce the general availability of OpenFunction 1.0.0.
In this update, we have continued our commitment to providing developers with more flexible and powerful tools, and have added some new feature. This release integrates WasmEdge to support Wasm functions; we have also enhanced the CI/CD functionality of OpenFunction to provide relatively complete end-to-end CI/CD functionality; and we have added the ability to build an image of a function or application directly from local code in this release, making it easier for developers to publish and deploy their code.
We have optimized OpenFunction’s performance and stability and fixed bugs to improve the user experience.
The following introduces the major updates.
Integrate WasmEdge to support Wasm Functions
WasmEdge is a lightweight, high-performance, and scalable WebAssembly runtime for cloud-native, edge, and decentralized applications. It powers serverless apps, embedded functions, microservices, smart contracts, and IoT devices.
OpenFunction now supports building and running wasm functions with WasmEdge as the workload runtime. WasmEdge has been an alternative container runtime of Docker, Containerd, and CRI-O.
Create a wasm function
cat <<EOF | kubectl apply -f -
apiVersion: core.openfunction.io/v1beta1
kind: Function
metadata:
name: wasmedge-http-server
spec:
workloadRuntime: wasmedge
image: openfunctiondev/wasmedge_http_server:0.1.0
imageCredentials:
name: push-secret
build:
dockerfile: Dockerfile
srcRepo:
revision: main
sourceSubPath: functions/knative/wasmedge/http-server
url: https://github.com/OpenFunction/samples
port: 8080
route:
rules:
- matches:
- path:
type: PathPrefix
value: /echo
serving:
runtime: knative
scaleOptions:
minReplicas: 0
template:
containers:
- command:
- /wasmedge_hyper_server.wasm
imagePullPolicy: IfNotPresent
livenessProbe:
initialDelaySeconds: 3
periodSeconds: 30
tcpSocket:
port: 8080
name: function
EOF
With the WasmEdge engine, developers can write and run functions using a variety of Wasm-enabled languages and development frameworks.
Please refer to the official documentation Wasm Functions.
Enhanced CI/CD
Previously users can use OpenFunction to build function or application source code into container images, and then the system deploys the built image directly to the underlying sync/async Serverless runtime without user intervention.
But OpenFunction can neither rebuild the image and then redeploy it whenever the function or application source code changes nor redeploy the image whenever this image changes (When the image is built and pushed manually or in another function)
Starting from v1.0.0, OpenFunction adds the ability to detect source code or image changes and then rebuild and/or redeploy the newly built image in a new component called Revision Controller. The Revision Controller is able to:
- Detect source code changes in GitHub, GitLab or Gitee, and then rebuild and redeploy the new built image whenever the source code changes.
- Detect the bundle container image (image containing the source code) changes, then rebuild and redeploy the new built image whenever the bundle image changes.
- Detect the function or application image changes, then redeploy the new image whenever the function or application image changes.
The enhanced CI/CD functionality ensures that the code runs efficiently in different environments, and users can have better control over the versions and code quality during the development and deployment process. This also provides a better user experience.
Please refer to the official documentation CI/CD.
Build functions from local source code
To build functions or applications from local source code, you’ll need to package your local source code into a container image and push this image to a container registry.
Suppose your source code is in the samples
directory, you can use the following Dockerfile
to build a source code bundle image.
FROM scratch
WORKDIR /
COPY samples samples/
Then you can build the source code bundle image like this:
docker build -t <your registry name>/sample-source-code:latest -f </path/to/the/dockerfile> .
docker push <your registry name>/sample-source-code:latest
It’s recommended to use the empty image
scratch
as the base image to build the source code bundle image, a non-empty base image may cause the source code copy to fail.
Unlike defining the spec.build.srcRepo.url
field for the git repo method, you’ll need to define the spec.build.srcRepo.bundleContainer.image
field instead.
apiVersion: core.openfunction.io/v1beta1
kind: Function
metadata:
name: logs-async-handler
spec:
build:
srcRepo:
bundleContainer:
image: openfunctiondev/sample-source-code:latest
sourceSubPath: "/samples/functions/async/logs-handler-function/"
The
sourceSubPath
is the absolute path of the source code in the source code bundle image.
Other enhancements
In addition to the major changes mentioned above, this release has the following changes and enhancements.
- OpenFunction
- The core v1alpha2 API was deprecated and removed
- Add sha256 to serving image
- Add information of build source to function status
- Bump shipwright to v0.11.0, knative to v0.32.0, dapr to v1.8.3, and go to 1.18
- functions-framework-java released version 1.0.0
- Support multiple functions in one pod
- Support for automatic publishing
- Builder
- Support multiple functions in one pod
- Update the default java framework version to 1.0.0
- revision-controller released version 1.0.0
These are the main feature changes in OpenFunction v1.0.0 and we would like to thank all contributors for their contributions. If you are looking for an efficient and flexible cloud-native function development platform, OpenFunction v1.0.0 is the perfect choice for you.
For more details and documentation, please visit our website and GitHub repo.
Announcing OpenFunction 0.8.0: Speed up function launching with Dapr Proxy
One of the unique features of OpenFunction is its simple integration with various backend services (BaaS) through Dapr. Currently, OpenFunction supports Dapr pub/sub and bindings building blocks, and more will be added in the future.
In OpenFunction v0.7.0 and versions prior to v0.7.0, OpenFunction integrates with BaaS by injecting a dapr sidecar container into each function instance’s pod, which leads to the following problems:
- The entire function instance’s launch time is slowed down by the launching of the dapr sidecar container.
- The dapr sidecar container may consume more resources than the function container itself.
To address the problems above, OpenFunction introduces the Dapr Standalone Mode
in v0.8.0.
Dapr Standalone Mode
In Dapr standalone mode, one Dapr Proxy service
will be created for each function which is then shared by all instances of this function. This way, there is no need to launch a seperate Dapr sidecar container for each function instance anymore which reduces the function launching time significantly.
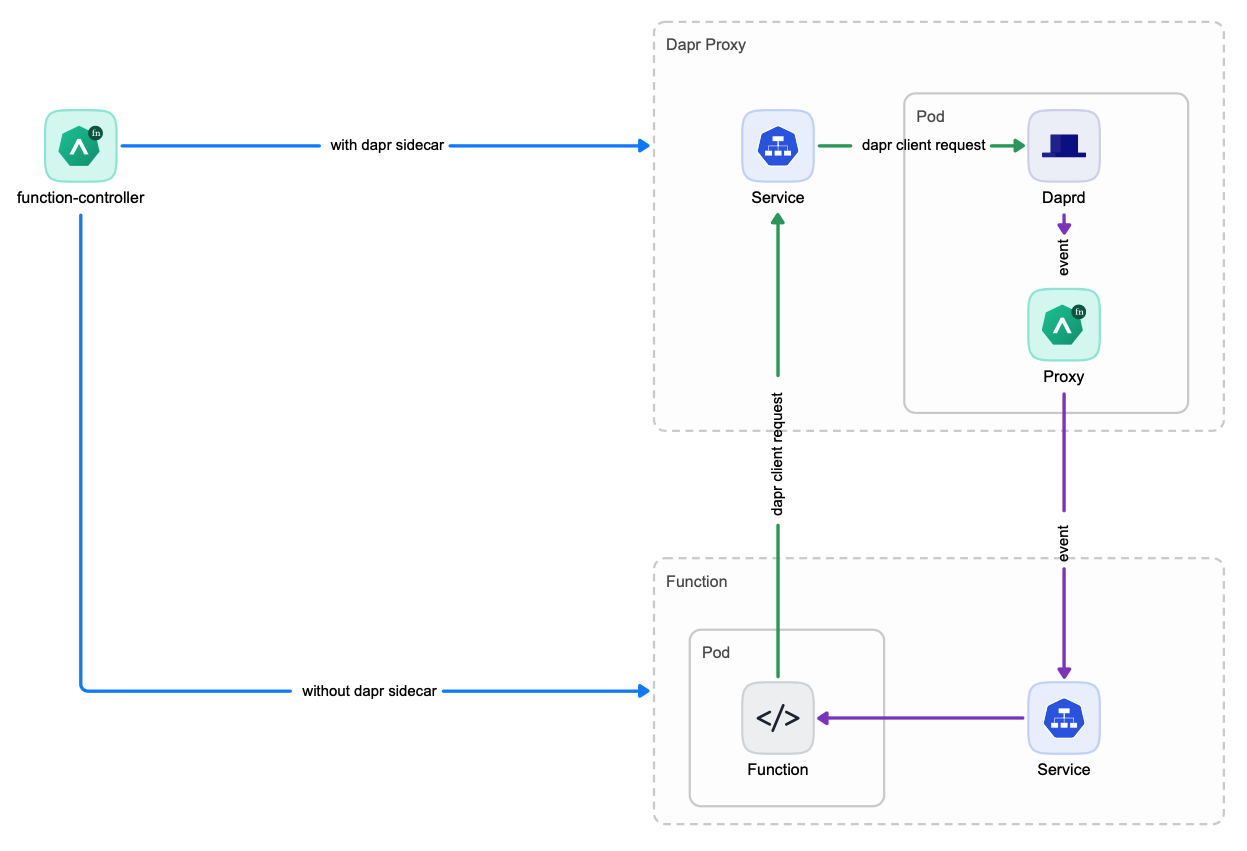
Choose the appropriate Dapr Service Mode
So now you’ve 2 options to integrate with BaaS:
Dapr Sidecar Mode
Dapr Standalone Mode
You can choose the appropriate Dapr Service Mode for your functions. The Dapr Standalone Mode
is the recommened and default mode. You can use Dapr Sidecar Mode
if your function doesn’t scale frequently or you’ve difficulty to use the Dapr Standalone Mode
.
You can control how to integrate with BaaS with 2 flags, both can be set in function’s spec.serving.annotations
:
openfunction.io/enable-dapr
can be set totrue
orfalse
openfunction.io/dapr-service-mode
can be set tostandalone
orsidecar
- When
openfunction.io/enable-dapr
is set totrue
, users can choose theDapr Service Mode
by settingopenfunction.io/dapr-service-mode
tostandalone
orsidecar
. - When
openfunction.io/enable-dapr
is set tofalse
, the value ofopenfunction.io/dapr-service-mode
will be ignored and neitherDapr Sidecar
norDapr Proxy Service
will be launched.
There’re default values for both of these two flags if they’re not set.
- The value of
openfunction.io/enable-dapr
will be set totrue
if it’s not defined inspec.serving.annotations
and the function definition contains eitherspec.serving.inputs
orspec.serving.outputs
. Otherwise it will be set tofalse
. - The default value of
openfunction.io/dapr-service-mode
isstandalone
if not set.
Below you can find a function example to set these two flags:
apiVersion: core.openfunction.io/v1beta1
kind: Function
metadata:
name: cron-input-kafka-output
spec:
...
serving:
annotations:
openfunction.io/enable-dapr: "true"
openfunction.io/dapr-service-mode: "standalone"
template:
containers:
- name: function # DO NOT change this
imagePullPolicy: IfNotPresent
runtime: "async"
inputs:
- name: cron
component: cron
outputs:
- name: sample
component: kafka-server
operation: "create"
...
Here you can find more information about OpenFunction v0.8.0.
Announcing General Availability of OpenFunction 0.7.0
OpenFunction is a cloud-native open-source FaaS (Function as a Service) platform aiming to let you focus on your business logic only. Today, we are thrilled to announce the general availability of OpenFunction 0.7.0.
Thanks to your contributions and feedback, OpenFunction 0.7.0 brings more exciting features like OpenFunction Gateway, async and sync functions of Java and Node.js, and Helm installation. Meantime, OpenFunction dependencies have been upgraded for better user experience.
OpenFunction Gateway
Starting from OpenFunction 0.5.0, OpenFunction uses Kubernetes Ingress to provide a unified entry for sync functions, and an Nginx ingress controller must be installed.
With the maturity of the Kubernetes Gateway API, we decided to implement OpenFunction Gateway based on the Kubernetes Gateway API to replace the previous ingress-based domain method in OpenFunction 0.7.0.
OpenFunction Gateway provides a more powerful and more flexible function gateway, including the following features:
Enable users to switch to any gateway implementations that support Kubernetes Gateway API such as Contour, Istio, Apache APISIX, Envoy Gateway (in the future) and more in an easier and vendor-neutral way.
Users can choose to install a default gateway implementation (Contour) and then define a new
gateway.networking.k8s.io
or use any existing gateway implementations in their environment and then reference an existinggateway.networking.k8s.io
.Allow users to customize their own function access pattern like
hostTemplate: "{{.Name}}.{{.Namespace}}.{{.Domain}}"
for host-based access.Allow users to customize their own function access pattern like
pathTemplate: "{{.Namespace}}/{{.Name}}"
for path-based access.Allow users to customize each function’s route rules (host-based, path-based or both) in the function definition and default route rules are provided for each function if there’re no customized route rules defined.
Access functions from outside of the cluster. You only need to configure a valid domain name in OpenFunction Gateway. Both Magic DNS and Real DNS are supported.
Send traffic to Knative service revisions directly without going through Knative’s own gateway anymore. You will need only OpenFunction Gateway since OpenFunction 0.7.0 to access OpenFunction sync functions, and you can ignore Knative’s domain config errors if you do not need to access Knative service directly.
In upcoming releases, OpenFunction will support traffic splitting between function revisions.
Support for multiple programming languages
The OpenFunction community has been striving to support more programming languages:
Go
functions-framework-go 0.4.0 supports the ability to define multiple sub-functions in one function and call the sub-functions through different Paths and Methods.
Java
functions-framework-java supports async and sync functions.
Node.js
functions-framework-nodejs 0.5.0 supports async and sync functions, and the async functions can be triggered by the sync functions.
OpenFunction will introduce more features in functions-framework-nodejs 0.6.0, such as add-ons and integration with SkyWalking.
OpenFunction will support more programming languages, such as Python and Dotnet.
Use Helm to install OpenFunction and its dependencies
Previously, we use CLI to install OpenFunction and its dependencies. Now, this method has been depreciated.
In OpenFunction 0.7.0., we use Helm to install OpenFunction and its dependencies, which is more cloud-native and solves the problem that some users are unable to access Google Container Registry (gcr.io).
TL;DR
helm repo add openfunction https://openfunction.github.io/charts/
helm repo update
helm install openfunction openfunction/openfunction -n openfunction --create-namespace
Upgrade dependencies
Components | OpenFunction 0.6.0 | OpenFunction 0.7.0 |
---|---|---|
Knative Serving | 1.0.1 | 1.3.2 |
Dapr | 1.5.1 | 1.8.3 |
Keda | 2.4.0 | 2.7.1 |
Shipwright | 0.6.1 | 0.10.0 |
Tekton Pipelines | 0.30.0 | 0.37.0 |
Announcing OpenFunction 0.6.0: FaaS observability, HTTP trigger, and more
OpenFunction is a cloud-native open source FaaS platform aiming to let you focus on your business logic. The OpenFunction community has been hard at work over the past several months preparing for the OpenFunction 0.6.0 release. Today, we proudly announce OpenFunction 0.6.0 is officially available. Thanks to the community for helping drive the new features, enhancements, and bug fixes.
OpenFunction 0.6.0 brings notable features including function plugin, distributed tracing for functions, control autoscaling behavior, HTTP trigger to async function, etc. Meanwhile, asynchronous runtime definition has also been refactored. The core API has been upgraded from v1alpha1 to v1beta1.
Distributed tracing for serverless functions
When trying to understand and diagnose the distributed systems and microservices, one of the most effective methods is the stack trace. Distributed tracing provides a holistic view of the way that messages flow and distributed transaction monitoring for Serverless functions. The OpenFunction team collaborates with the Apache SkyWalking community to add FaaS observability which allows you to visualize function dependencies and track function invocations on SkyWalking UI.
Going forward, OpenFunction will add more capabilities for serverless functions in logging, metrics, and tracing. You will be able to use Apache SkyWalking and OpenFunction to set up a full-stack APM for your serverless workloads out-of-the-box. Moreover, OpenFunction will support OpenTelemetry that allows you to leverage Jaeger or Zipkin as an alternative.
Support Dapr pub/sub and bindings
Dapr bindings allows you to trigger your applications or services with events coming in from external systems, or interface with external systems. OpenFunction 0.6.0 adds Dapr output bindings to its synchronous functions which enables HTTP triggers for asynchronous functions. For example, synchronous functions backed by the Knative runtime can now interact with middlewares defined by Dapr output binding or pub/sub, and an asynchronous function will be triggered by the events sent from the synchronous function. See this guide for the quickstart sample.
Asynchronous function introduces Dapr pub/sub to provide a platform-agnostic API to send and receive messages. A typical use case is that you can leverage synchronous functions to receive an event in plain JSON or Cloud Events format, and then send the received event to a Dapr output binding or pub/sub component, most likely a message queue (e.g. Kafka, NATS Streaming, GCP PubSub). Finally, the asynchronous function could be triggered from the message queue. See this guide for the quickstart sample.
Control autoscaling behavior for functions
OpenFunction 0.6.0 integrates KEDA ScaledObject spec which is used to define how KEDA should scale your application and what the triggers are. You just need to define the lower and upper bound in the function CRD without changing your code.
Meanwhile, the OpenFunction community is also developing the ability to control the concurrency and the number of simultaneous requests, which inherits the definition from Dapr and Knative. A typical use case in distributed computing is to only allow for a given number of requests to execute concurrently. You will be able to control how many requests and events will invoke your application simultaneously. This feature will be totally supported in the next release, stay tune! If you are interested in this feature, check out the active discussion for detailed context.
Learn by doing
Benjamin Huo, the founder of OpenFunction, has presented two typical use cases of OpenFunction 0.6.0 in Dapr community meeting:
- HTTP trigger for asynchronous functions with OpenFunction and Kafka
- Elastic Kubernetes log alerts with OpenFunction and Kafka
Watch this video and follow with the hands-on guides to practice.
You can learn more about OpenFunction 0.6.0 from release notes. Get started with OpenFunction by following the Quick Start and samples.
Release v0.4.0
@wanjunlei wanjunlei released this on 2021.10.19
Release: v0.4.0
Release notes:
Features
Enhancement
- Update dependent Dapr version to v1.3.1. #123
- Update dependent Tekton pipeline version to 0.28.1. #131
- Update dependent Knative serving version to 0.26.0. #131
- Update dependent Shipwright build version to 0.6.0. #131
- Update go version to 1.16. #131
- Now Function supports environment variables with commas. #131
Fixes
- Fix bug rerun serving failed. #132
Docs
- Use installation script to deploy OpenFunction and deprecate the installation guide. #122
OpenFunction/website
- Add OpenFunction Website. #1
OpenFunction/cli
- Add OpenFunction CLI. #11
OpenFunction/builder
- Upgrade the functions-framework-go from v0.0.0-20210628081257-4137e46a99a6 to v0.0.0-20210922063920-81a7b2951b8a. #17
- Add Ruby builder. #11
OpenFunction/functions-framework-go
- Supports multiple input sources && Replace int return with ctx.Return. #13
OpenFunction/functions-framework-nodejs
- Support OpenFunction type function. #7
OpenFunction/events-handlers
- Add handler functions. #7
Thanks
Thanks to these contributors who contributed to v0.4.0!
@wanjunlei @benjaminhuo @tpiperatgod @linxuyalun @penghuima @lizzzcai
Release v0.3.1
@wanjunlei wanjunlei released this on 2021.8.27
Release: v0.3.1
Release notes:
- Delete the old serving CR only after the new serving CR is running. #107
Release v0.3.0
@tpiperatgod tpiperatgod released this on 2021.8.19
Release: v0.3.0
Release notes:
- Add OpenFunction Events: OpenFunction’s own event management framework. #78 #83 #89 #90 #99 #100 @tpiperatgod
- Support using Shipwright as Builder backend to build functions or apps. #82 #95 @wanjunlei
- Build and serving can be launched separately now. #82 #95 @wanjunlei
- Support running an application (container image) as a serverless workload directly. #82 #95 @wanjunlei
Release v0.2.0
@benjaminhuo benjaminhuo released this on 2021.5.17
Release: v0.2.0
Release notes:
- Support OpenFunctionAsync serving runtime(backed by Dapr + KEDA + Deployment/Job)
- Functions framework supports async function now
- Customized go function framework & builders for both Knative and OpenFunctionAsync serving runtime
Release v0.1.0
@benjaminhuo benjaminhuo released this on 2021.5.17
Release: v0.1.0
Release notes:
- Add Function, Builder, and Serving CRDs and corresponding controllers
- Support using existing function framework & buildpacks such as Google Cloud Functions to build functions
- Support Tekton and Cloud Native Buildpacks as Builder backend
- Support Knative as Serving backend
- Optimize and localize existing function framework & buildpacks